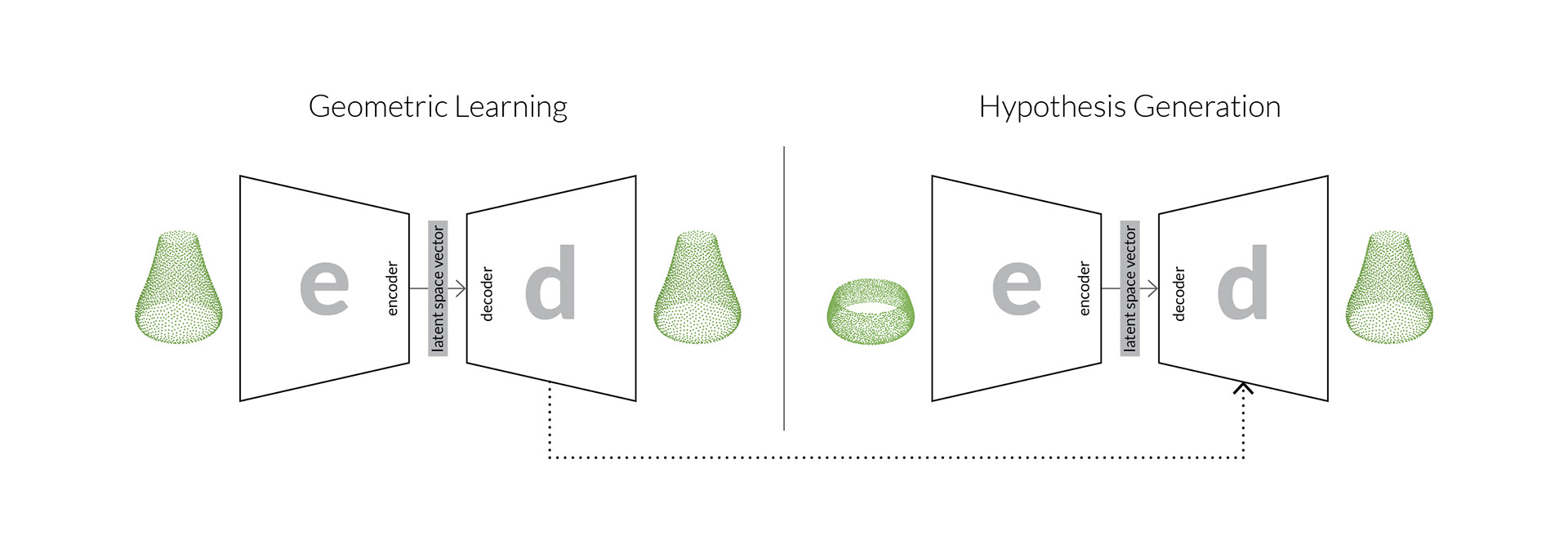
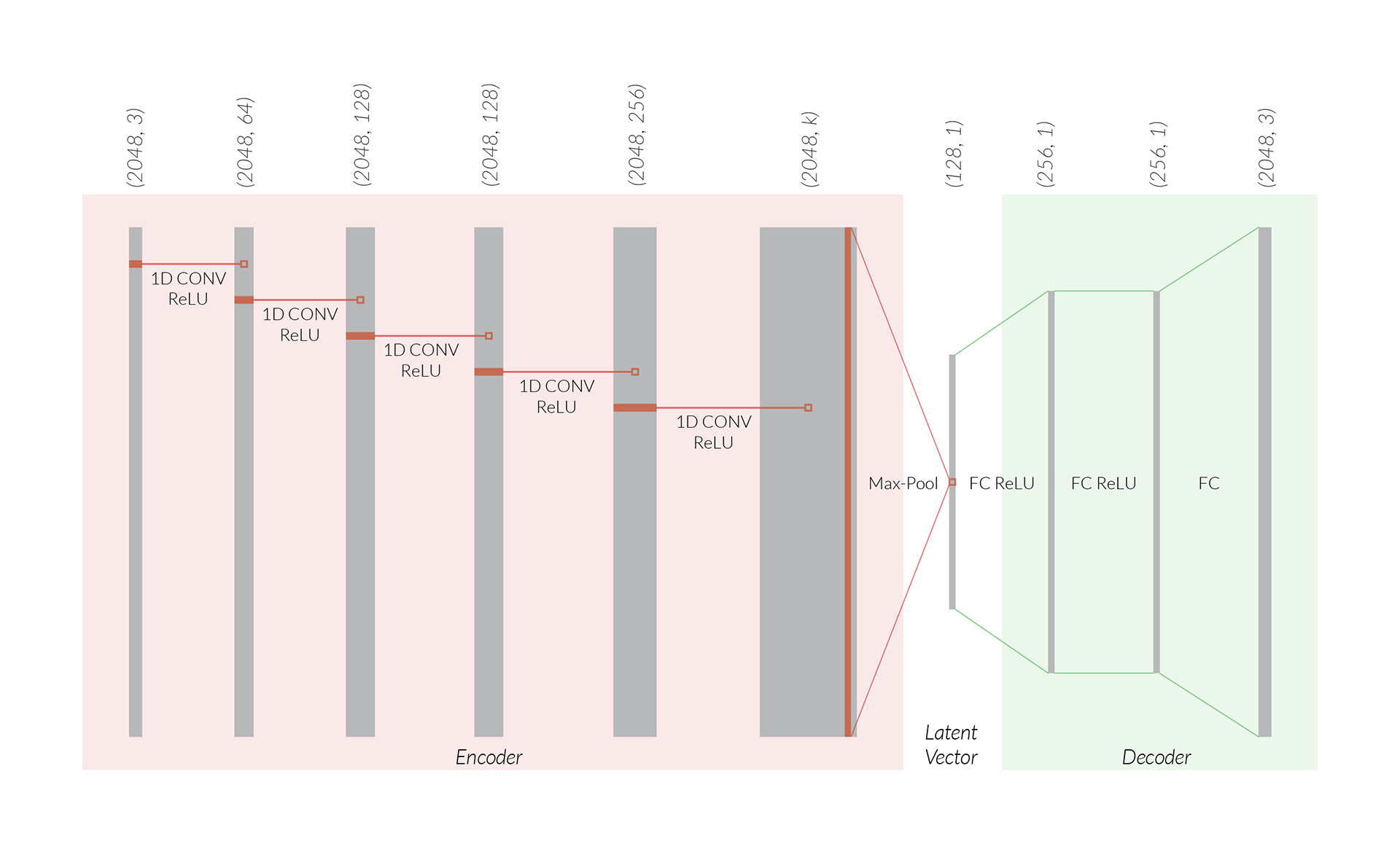
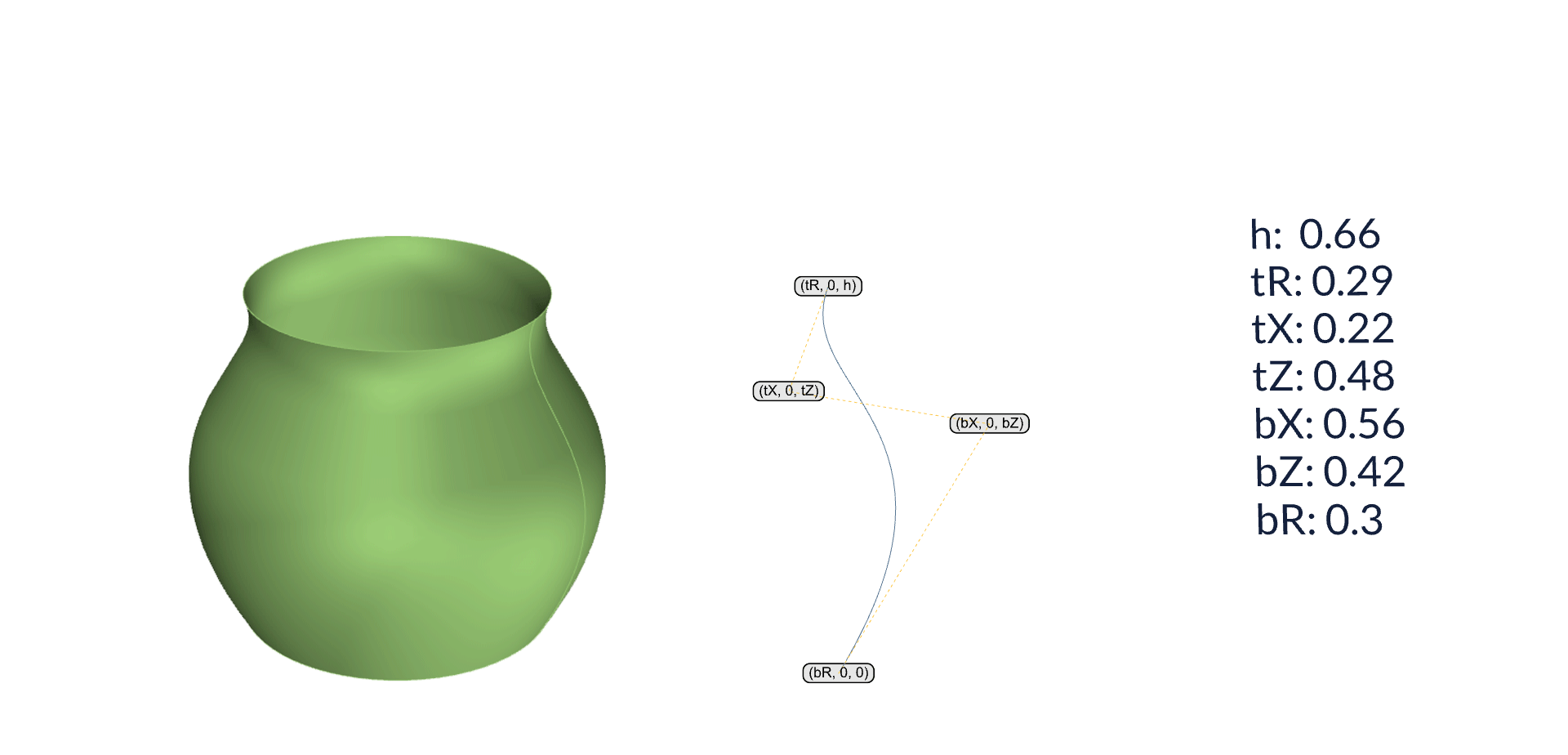
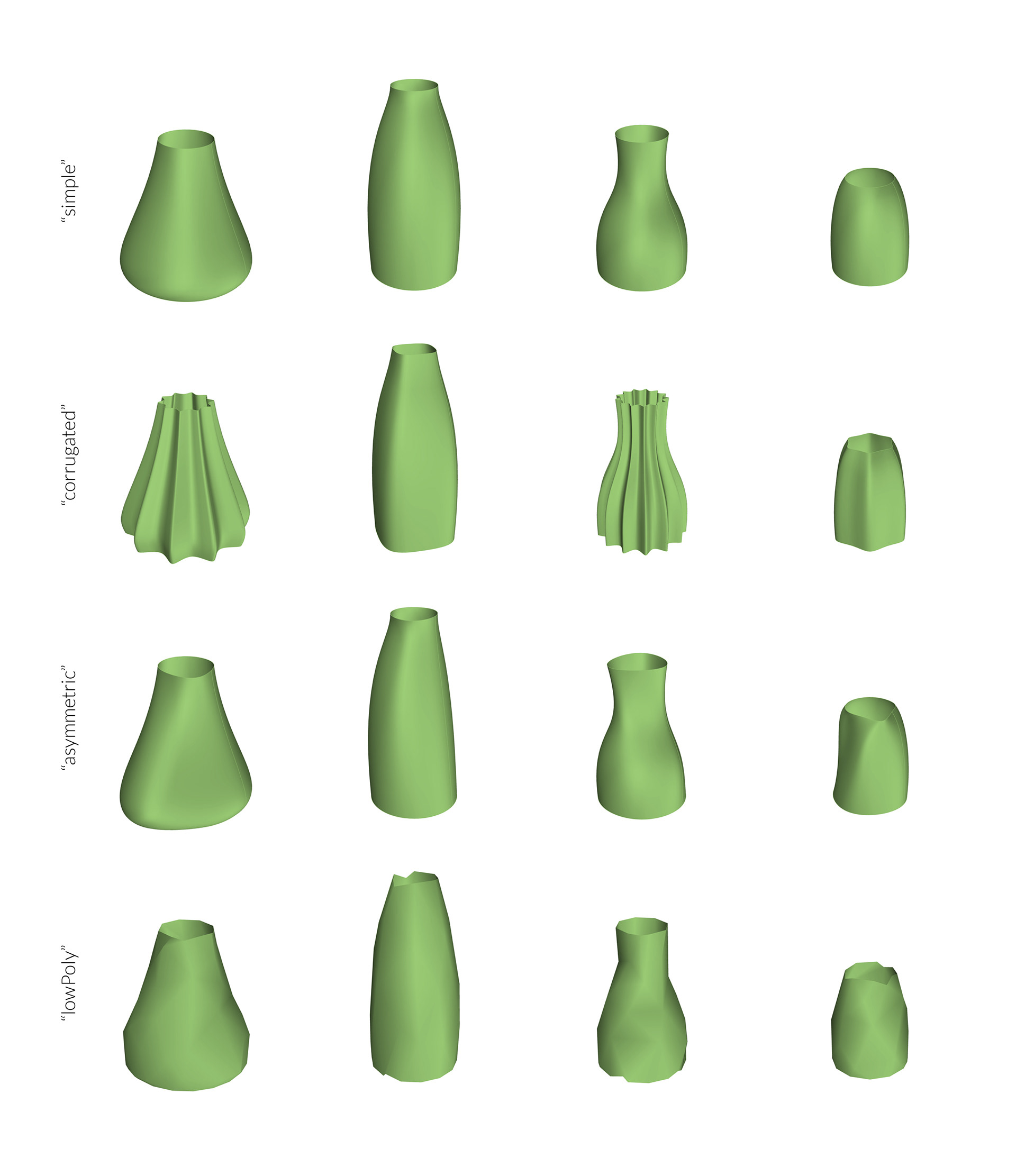
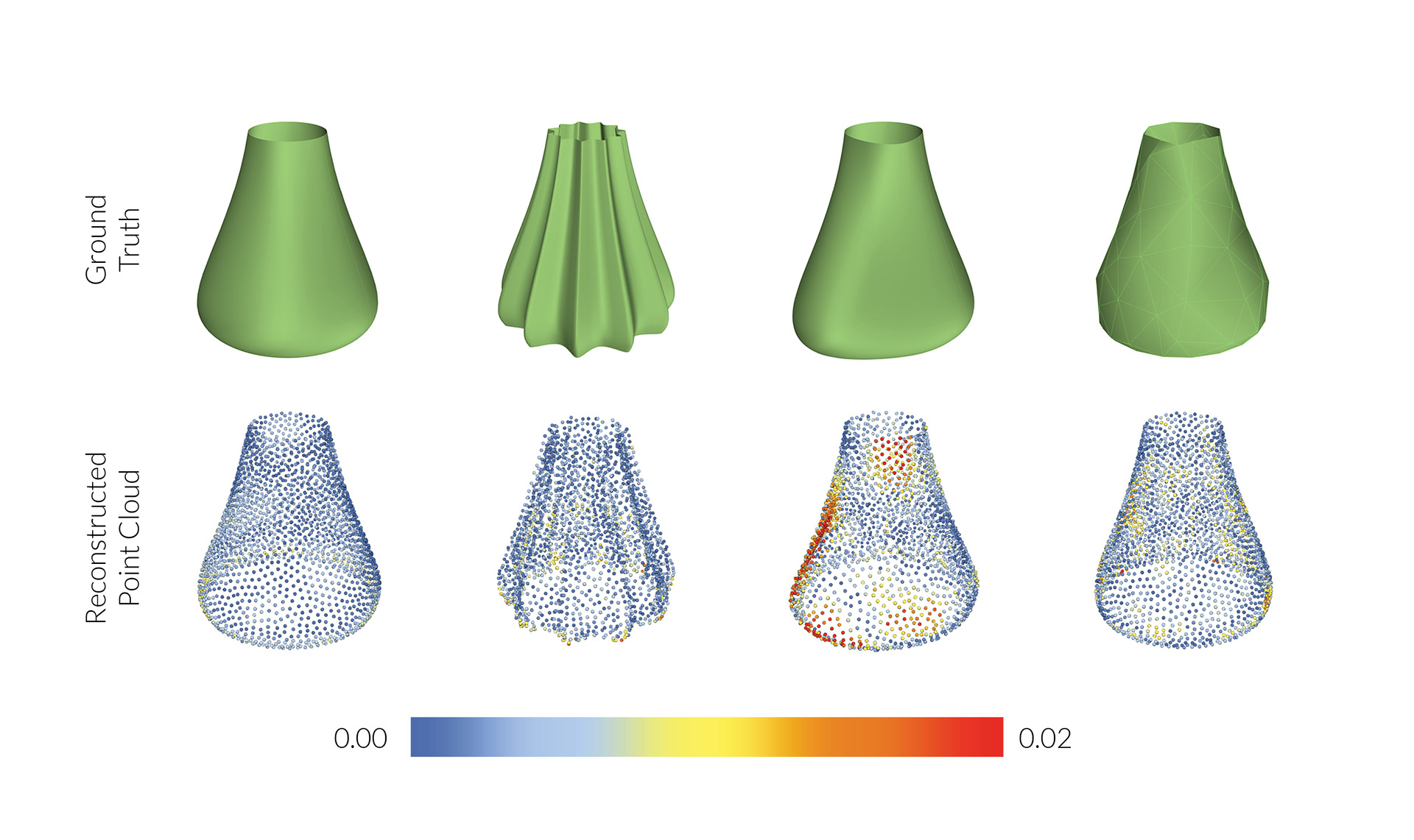
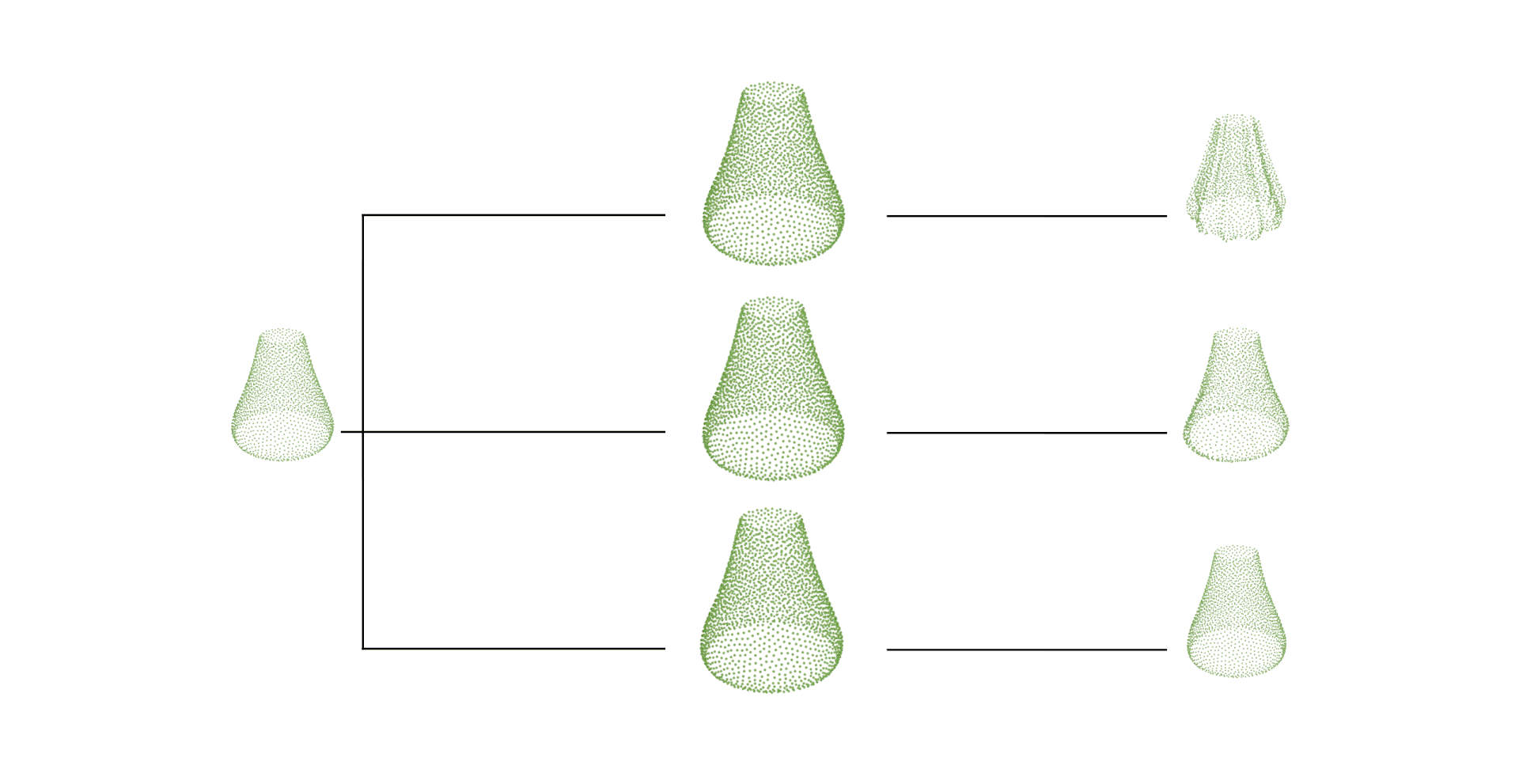
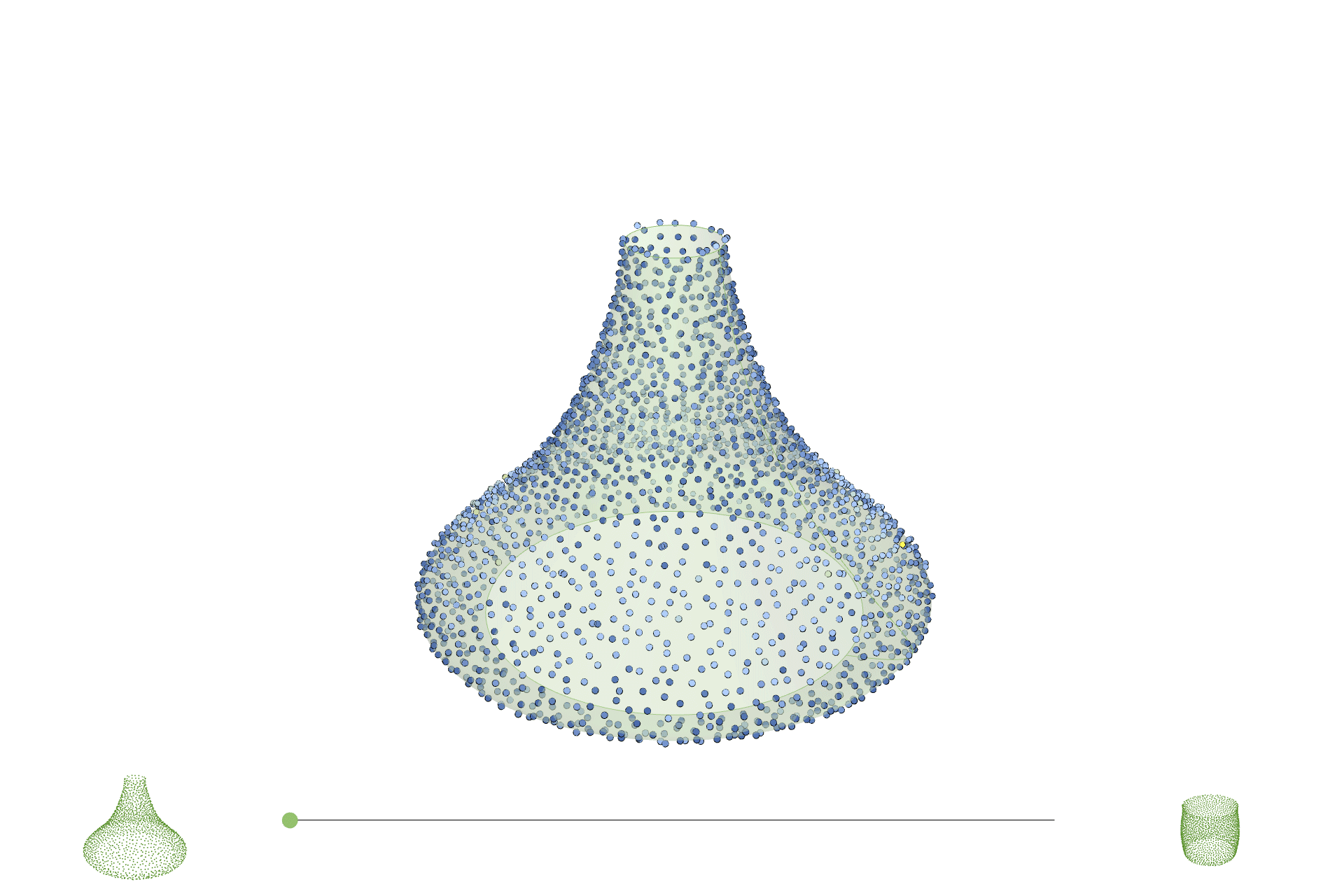
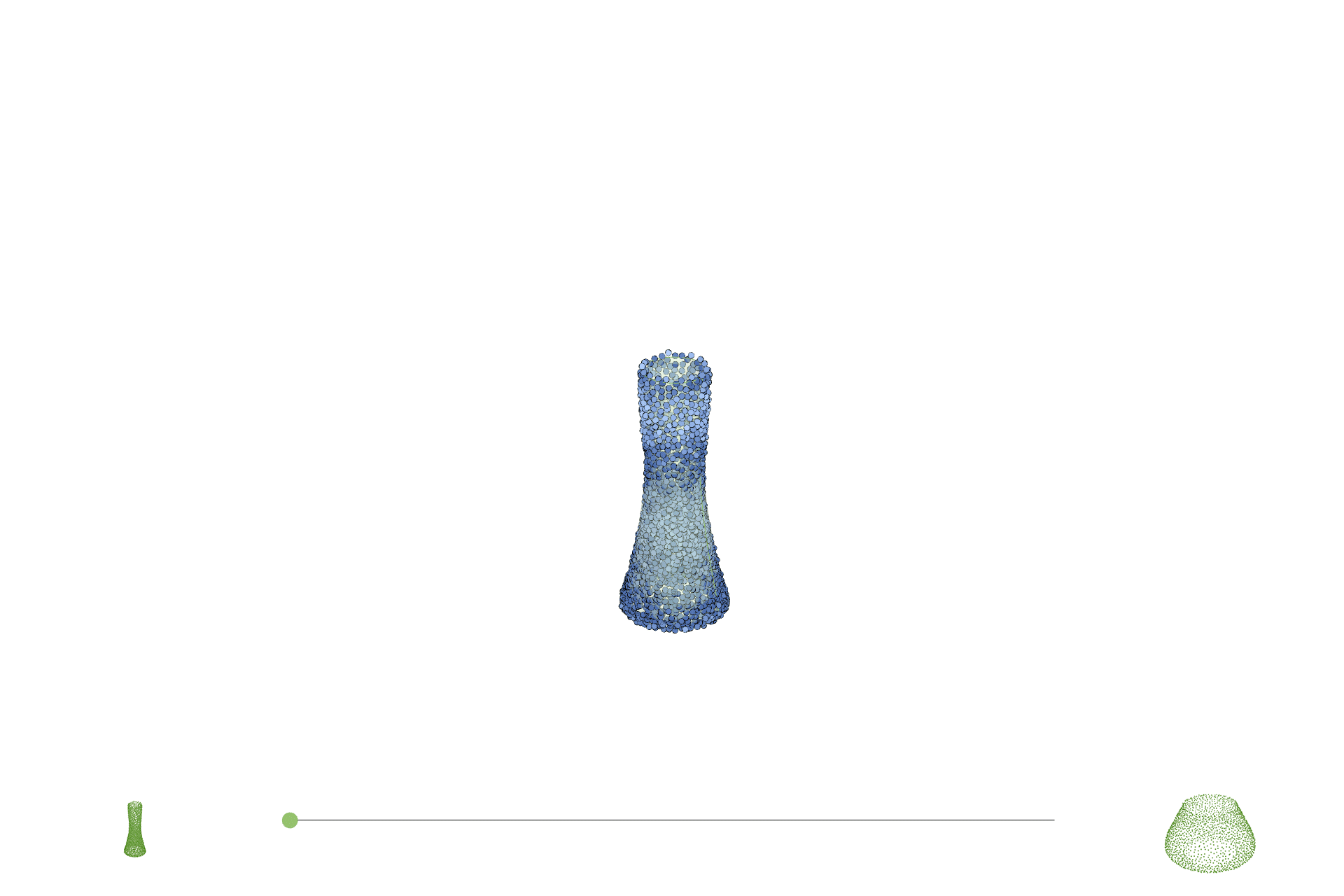
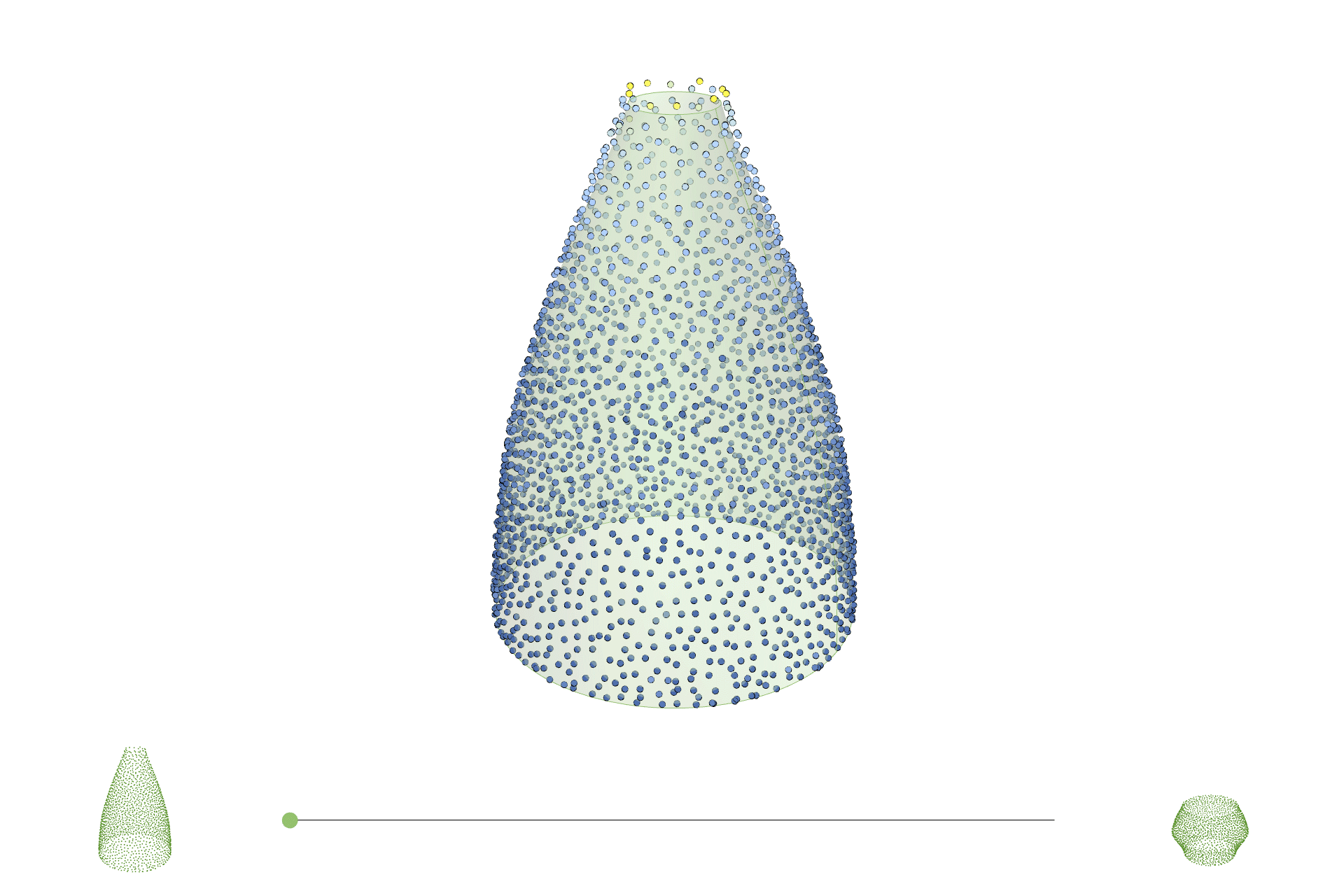
This research investigates the potential of learning-based models, particularly Autoencoder (AE) networks, in enhancing creative inquiries within computer-aided design (CAD) systems. It challenges the conventional role of CAD as mere task executors, aiming to develop a system that offers reciprocal interaction and design suggestion generation. The study explores their capability to represent and learn various design approaches and intentions in a lower-dimensional latent space by training AE networks on problem-specific datasets. This work represents the first stage in understanding the reconstruction capabilities of AE networks through synthetic datasets tailored to specific design problems. Collaborators: Shakthi Suresh, Chris McComb